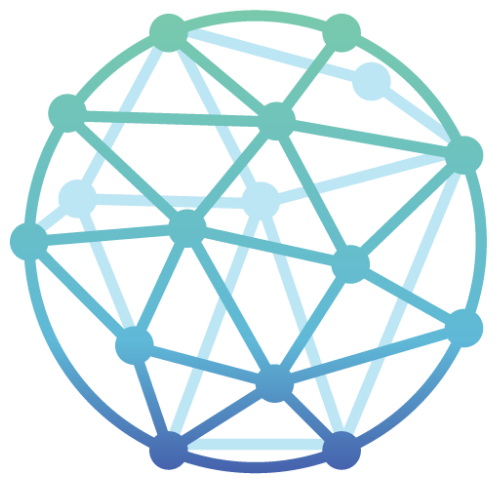
We teach simple quant trading techniques that anyone can apply.
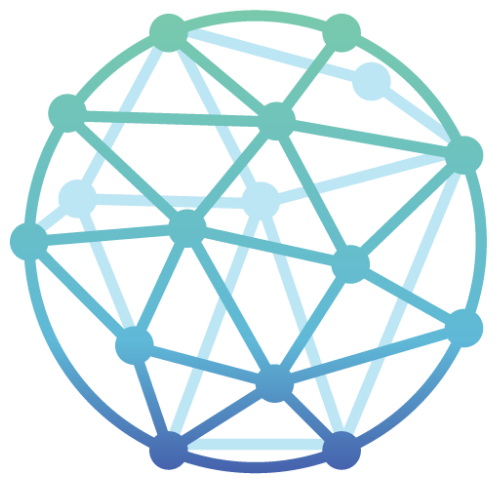
Trade Like a Quant Bootcamp is currently closed for enrolment.
Trade Like a Quant Bootcamp will open again for enrolments Q3 2024.
Join the waitlist for bootcamp by subscribing to our newsletter.
Hi! We’re James & Kris
We guide the Robot Wealth community with the trading approach that, between us, we’ve used for over 30 years.
We’re here to help you fast-track your systematic trading portfolio and your quant trading skills.
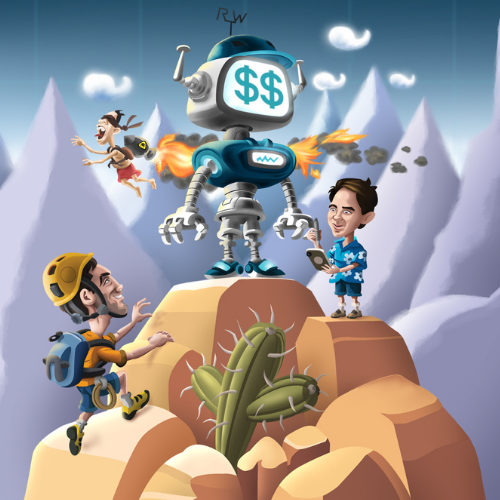
Quant Trading Bootcamps
We run quant trading Bootcamps – open to the public – several times a year.
In Bootcamp, you will learn a simple, high-probability, quantitative approach to trading that can work for you, the non-professional trader. You will focus on simple strategies based on economically-sensible, quantifiable market edges that a part-time trader can manage.
Bootcamp enrollment is currently CLOSED. Join the waiting list for the next edition here.
AS SEEN ON
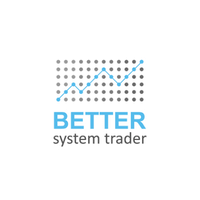
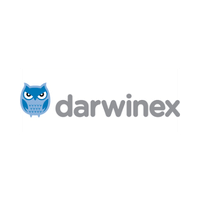
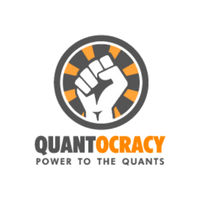
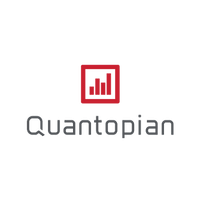
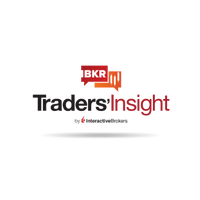
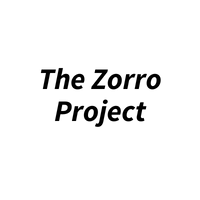
Free stuff to get you started
LATEST BLOG POSTS
SOME TWITTER THREAD GOLD
FOLLOW US ON SOCIAL
RW Trading Community
RW Pro is a community of professional and part-time traders with whom we share our trades, research, data, technology, and know-how (as well as a healthy smattering of dad jokes).
COMMUNITY
Private Slack Workspace
Weekly webinars
Q&A sessions
Research & Collaboration
Collaborative research tools
Curated data sets
Database of market inefficiencies
Training
Watch & participate in our real-time R&D
Access all past & future bootcamps
All training material we’ve ever published
Trading
RW Portfolio API
Research & code for RW strategies
Tools for trading the RW Portfolio