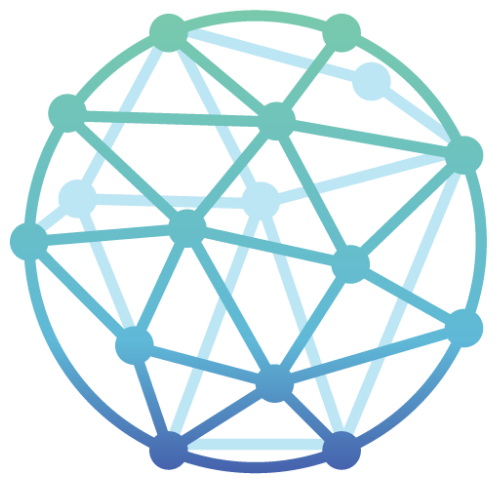
We teach simple quant trading techniques that anyone can apply.
Hi! We’re James & Kris
We run our own trading operation, wear Hawaiin shirts, and guide the Robot Wealth community with the trading approach that, between us, we’ve used for over 30 years.
If you’re a solo trader, our free newsletter will help you fast-track your systematic trading portfolio and your quant trading skills.
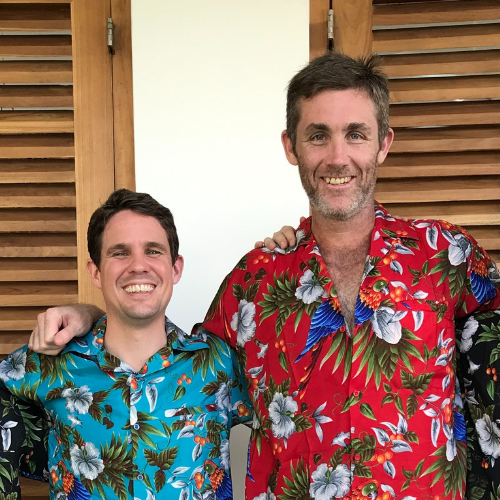
Join the newsletter and we’ll also send you a 63-page case study on how Kris left an engineering career for professional trading and later built Robot Wealth.
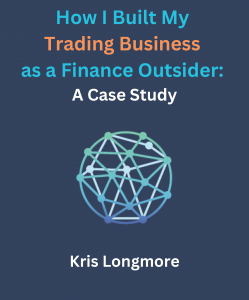
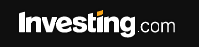
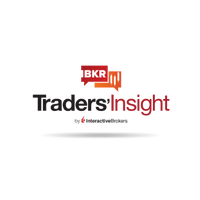
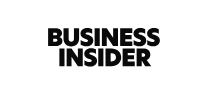
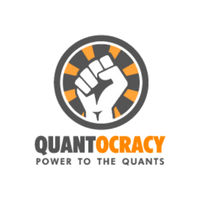
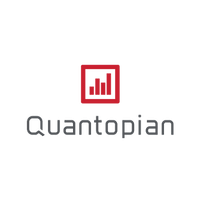
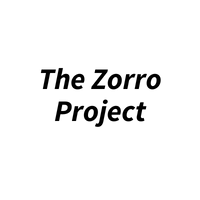
Quant Trading Bootcamps
We run quant trading Bootcamps designed to help you become brilliant at the basics of quant trading.
In Bootcamp, you will learn a simple, high-probability, quantitative approach to trading that can work for you, the non-professional trader. You will focus on simple strategies based on economically-sensible, quantifiable market edges that a part-time trader can manage.
Find out more about our Bootcamp program here.
Free stuff to get you started
LATEST BLOG POSTS
SOME TWITTER THREAD GOLD
FOLLOW US ON SOCIAL
RW Trading Community
RW Pro is a community of professional and part-time traders with whom we share our trades, research, data, technology, and know-how (as well as a healthy smattering of dad jokes).
COMMUNITY
Private Slack Workspace
Weekly webinars
Q&A sessions
Research & Collaboration
Collaborative research tools
Curated data sets
Database of market inefficiencies
Training
Watch & participate in our real-time R&D
Access all past & future bootcamps
All training material we’ve ever published
Trading
RW Portfolio API
Research & code for RW strategies
Tools for trading the RW Portfolio